Harnessing the Power of Agriculture Datasets for Machine Learning
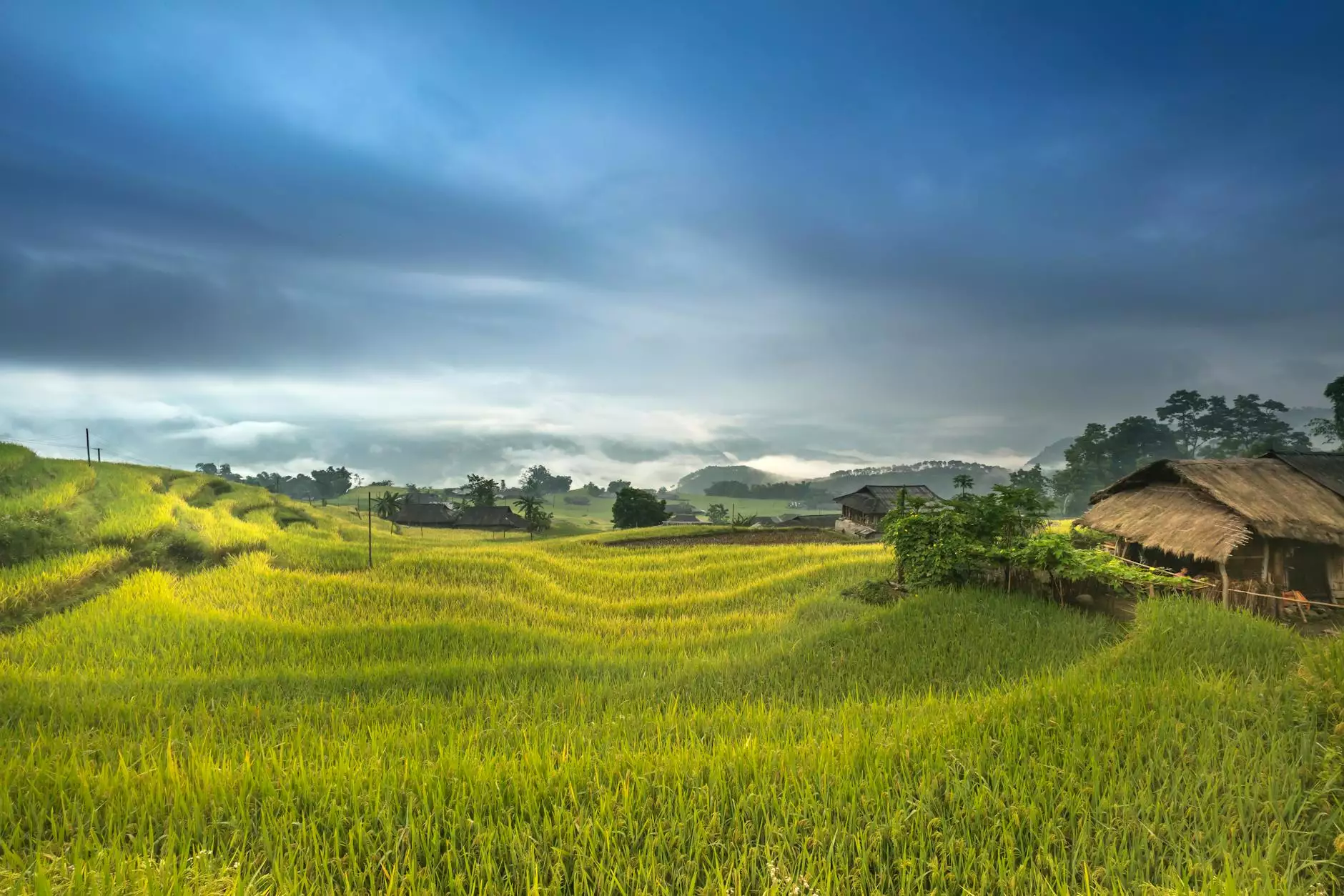
Agriculture has always been a cornerstone of civilization, and as we advance further into the 21st century, the integration of technology within this sector has become paramount. One such technological marvel is the application of machine learning techniques, which rely heavily on data. In this context, agriculture datasets for machine learning emerge as invaluable resources that can transform traditional practices into modern, efficient systems.
The Importance of Data in Modern Agriculture
Data plays a critical role in contemporary agriculture. With the advent of precision farming and smart agriculture technologies, farmers have access to an overwhelming amount of data. This data ranges from soil composition, weather patterns, crop yields, pest infestations, and much more. The challenge lies not in data collection but in harnessing this information to make informed decisions.
Understanding Agriculture Datasets
Agriculture datasets consist of structured and unstructured data collected from various sources, including:
- Satellite Imagery: High-resolution images can provide insights into vegetation health, soil moisture levels, and land use.
- Crop Yields: Historical yield data enables modeling and predicting future outputs.
- Weather Data: Meteorological data is crucial for understanding climatic impacts on farming.
- Soil Health Metrics: Includes pH levels, nutrient content, and moisture levels that affect plant growth.
- Pest and Disease Records: Information on pest occurrences and disease outbreaks that can affect crop health.
Machine Learning Techniques in Agriculture
Implementing machine learning in agriculture involves the use of algorithms that analyze vast datasets to identify patterns and make predictions. The following are some prominent techniques:
- Predictive Analytics: Utilizes historical data to predict future trends in crop yields and growth conditions.
- Image Recognition: Machine learning models can be trained to recognize crop diseases or pests from images taken by drones or smartphones.
- Data Clustering: This technique groups similar datasets to help identify patterns in soil or crop health related to specific conditions.
- Decision Trees: These models assist farmers in making decisions based on multiple input features (such as weather or soil data).
- Neural Networks: Used for more complex pattern recognition and prediction tasks, especially in larger datasets.
Applications of Agriculture Datasets for Machine Learning
The applications of agriculture datasets for machine learning are vast and transformative:
1. Precision Farming
Precision farming utilizes machine learning algorithms to analyze data collected from the farm, allowing farmers to apply the right amounts of water, fertilizer, and pesticides at the right time and place. This leads to
Benefits:
- Resource Optimization: Maximizes resource efficiency, leading to cost savings.
- Enhanced Crop Yields: Data-driven decisions improve yield quality and quantity.
- Environmental Sustainability: Reduces the environmental impact of farming practices.
2. Crop Disease Prediction
Machine learning models can analyze historical data concerning weather conditions and past crop diseases to predict future outbreaks. By identifying the conditions under which diseases thrive, farmers can take preemptive actions.
Benefits:
- Early Detection: Enables early intervention, potentially saving entire crops.
- Targeted Treatment: Reduces the need for broad-spectrum pesticide use, promoting sustainability.
3. Yield Prediction
Yield prediction models help farmers anticipate their outputs based on various variables such as weather, soil health, and historical yield data. This allows for more informed financial and operational decisions.
Benefits:
- Financial Planning: Better forecasting aids in budgeting and investment.
- Market Readiness: Improves the ability to meet market demands more accurately.
4. Resource Management
Machine learning can optimize water usage and management based on data analytics from extensive datasets. By employing advanced irrigation systems that react in real-time to datasets, farmers achieve more efficient water usage.
Benefits:
- Water Conservation: Reduces wastage, conserves resources for future use.
- Cost Savings: Minimizes unnecessary expenditure on water resources.
Challenges in Utilizing Agriculture Datasets
While the benefits of using agriculture datasets for machine learning are evident, several challenges persist:
- Data Quality: Inaccurate or incomplete data can lead to poor predictive outcomes.
- Integration: Merging data from various sources can be complex and time-consuming.
- Skill Gap: There is a shortage of professionals who are skilled in both agriculture and data science.
- Cost of Technology: Initial investment in machine learning technologies can be high, posing a barrier for small-scale farmers.
The Future of Agriculture Datasets and Machine Learning
The future of agriculture is increasingly intertwined with the advancements in machine learning. As technology becomes more accessible, we can expect to see:
- Improved Data Collection: IoT devices and sensors will gather more precise and real-time data.
- Enhanced Analytical Tools: Software tools that make data analysis easier and more intuitive.
- Broader Adoption of AI: More farmers embracing AI and machine learning technologies in their operations.
- Collaboration across Industries: Partnerships between tech companies and agriculture will foster innovation.
Conclusion
In conclusion, the integration of agriculture datasets for machine learning presents an exciting frontier for the agricultural sector. By leveraging data, farmers can enhance efficiency, boost yields, and make sustainable decisions that benefit both the economy and the environment. The journey toward smarter agriculture is just beginning, and as technology evolves, so too will the opportunities for better practices and profitability.
Explore more about how machine learning can revolutionize your agricultural practices at Keymakr.
agriculture dataset for machine learning