Maximizing Business Efficiency with Production ML Systems
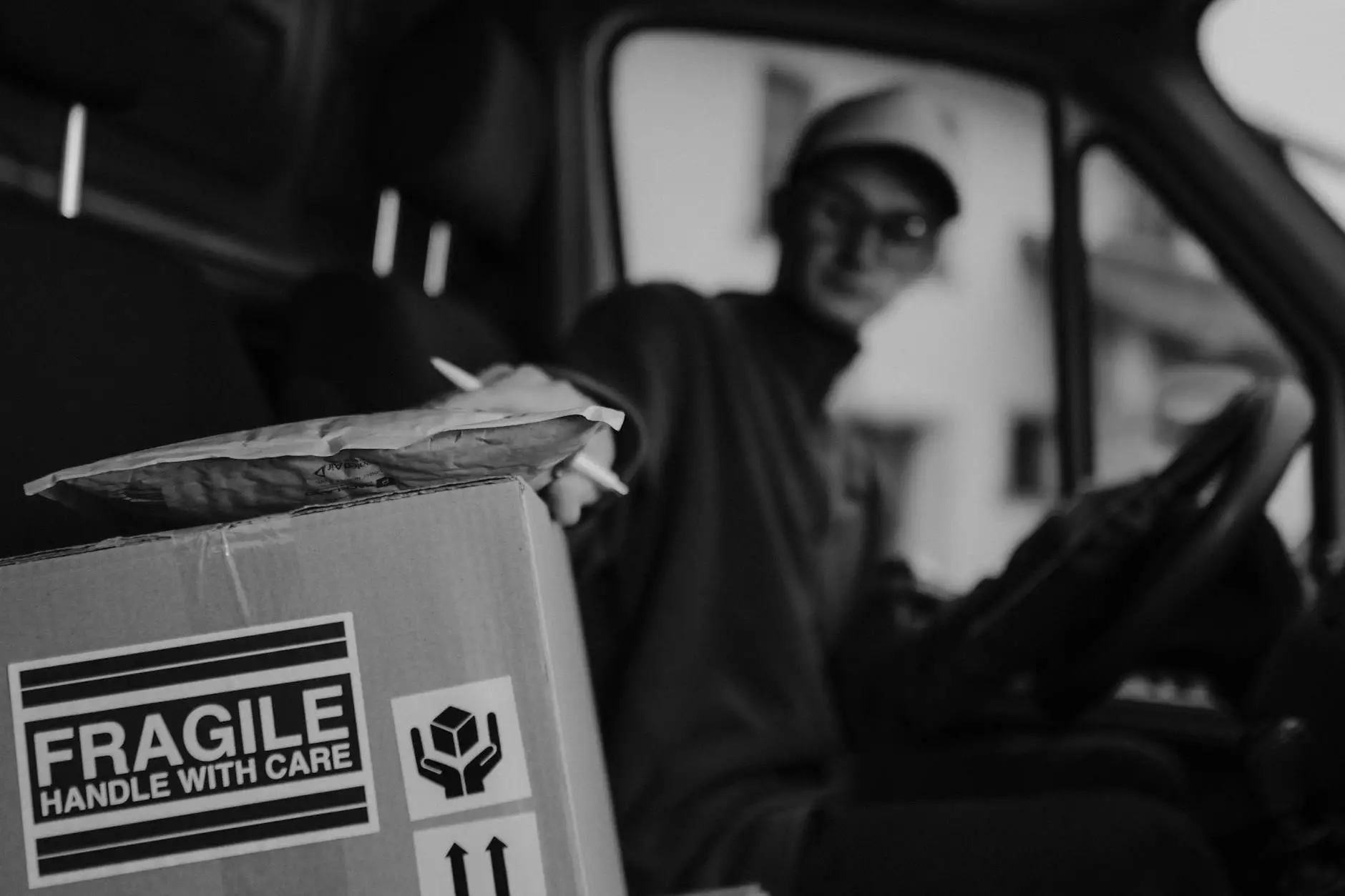
The modern business landscape is rapidly evolving, with technology at the forefront of this transformation. One of the most revolutionary advancements in recent years has been the emergence of production ML systems. These systems are changing the way companies operate, automating processes, enhancing data governance, and improving content management services. In this article, we will delve into the significance of production ML systems and how they can drastically improve your business operations.
Understanding Production ML Systems
Production machine learning (ML) systems refer to the deployment of machine learning models into a live production environment. These systems enable businesses to leverage data analysis, predictive analytics, and decision-making processes in real-time. By utilizing these systems, companies can increase their efficiency, reduce costs, and enhance customer experiences.
The Role of Production ML Systems in Business Process Automation
Business process automation (BPA) is fundamental in today’s fast-paced environment. By integrating production ML systems, organizations can automate repetitive tasks, facilitating smoother operations. Here are some key benefits of incorporating machine learning into your business processes:
- Increased Efficiency: Automation through ML reduces the time spent on manual data entry and processing.
- Enhanced Accuracy: Machine learning algorithms minimize human error, ensuring data integrity.
- Cost Savings: Automating processes can lower operational costs by reducing the need for extensive manpower.
- Scalability: ML systems can handle increased workloads without significant changes to infrastructure.
Data Governance Systems: Ensuring Compliance and Security
Incorporating production ML systems also enhances data governance. As businesses collect vast amounts of data, managing this data effectively becomes crucial. Here's how ML systems contribute to data governance:
- Data Quality: Machine learning algorithms can identify and correct data inconsistencies and errors.
- Regulatory Compliance: ML systems facilitate compliance with data regulations by automating data reporting and audits.
- Security Enhancements: Anomaly detection capabilities in ML can identify potential security breaches in real-time.
Optimizing Content Management Services with Production ML Systems
Effective content management is vital for businesses aiming to thrive in the digital sphere. Production ML systems can be a game-changer in how companies handle their content management services:
- Content Personalization: ML algorithms analyze user behavior, allowing businesses to tailor content to meet individual customer needs.
- Automated Tagging and Categorization: Machine learning can automate the process of tagging and categorizing content, improving searchability.
- Performance Analytics: ML provides deep insights into content performance, aiding in strategic decision-making.
Key Considerations for Implementing Production ML Systems
While the benefits of production ML systems are manifold, there are essential factors to consider before implementation:
- Data Infrastructure: Ensure that your data infrastructure can support ML deployment.
- Skillset: Assess the skills of your team regarding ML and data science. Training may be required.
- Integration: Choose ML tools and platforms that seamlessly integrate with existing systems.
- Continuous Monitoring: Once implemented, ML systems require ongoing monitoring and fine-tuning to maintain performance.
Case Studies: Success Stories of Production ML Systems
To illustrate the practical advantages of production ML systems, let’s examine some success stories from various industries:
1. Retail Sector
A major retail chain adopted production ML systems to analyze customer purchasing behaviors. By implementing predictive analytics, they were able to optimize inventory management, leading to a 20% reduction in stockout occurrences, which significantly improved customer satisfaction and increased revenue.
2. Financial Services
A leading financial institution utilized ML for fraud detection. By deploying a machine learning model, they detected suspicious transactions with greater accuracy, reducing fraudulent losses by 30% in just one year.
3. Healthcare
A healthcare provider integrated machine learning systems to enhance patient care. The system analyzed patient records and identified potential health risks, leading to proactive interventions that improved patient outcomes and reduced hospitalization rates.
Future of Production ML Systems in Business
The future of production ML systems is bright as more businesses recognize their potential. The increasing volume of data, combined with advancements in technology, is set to propel these systems even further. Companies will likely focus on:
- Enhanced AI Capabilities: Continued advancements in AI will allow for more sophisticated ML models.
- Greater Accessibility: As tools become more user-friendly, a wider range of businesses will implement ML solutions.
- Ethics and Governance: As the use of ML systems grows, so will the emphasis on ethical considerations and governance.
Conclusion
Production ML systems are not just a trend; they represent a fundamental shift in the approach organizations take to optimize their operations. By embracing these systems, businesses can automate processes, enhance data governance, and improve content management services. The result is greater efficiency, reduced costs, and ultimately, a stronger competitive edge in the marketplace. To stay ahead, it is crucial for companies to explore and implement production ML systems effectively, paving the way for a more productive and sustainable future.
For organizations looking to leverage production ML systems, Intalio.com offers expert solutions in content management, business process automation, and data governance. Explore how we can help you transform your business today.