The Future of Medical Data Annotation: Transforming Healthcare
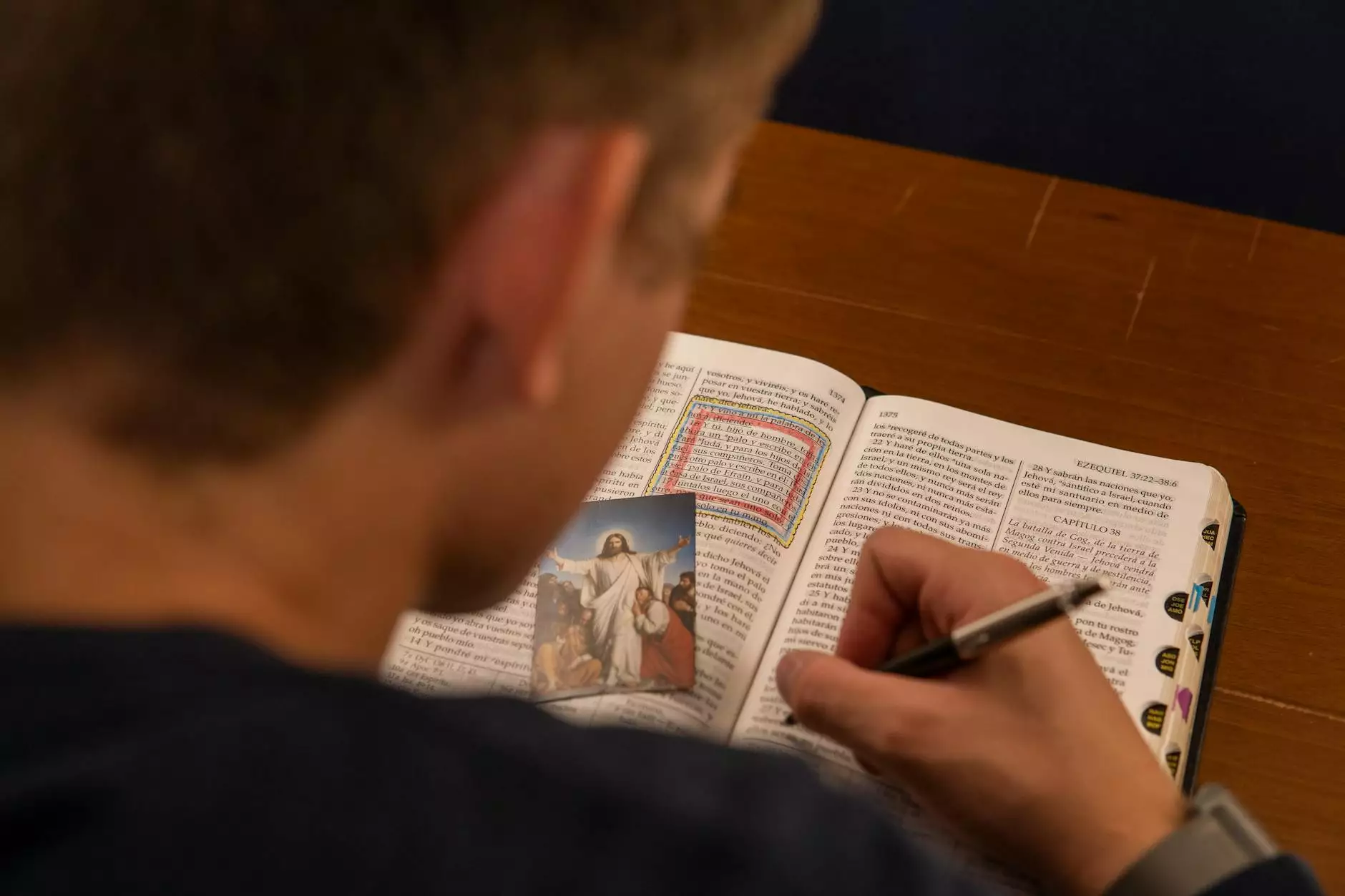
In today's fast-paced technological world, the role of medical data annotation has become increasingly crucial. As healthcare continues to evolve, the demand for accurate and meaningful data has surged. This article delves into the significance of medical data annotation, its applications, the technologies involved, and future trends that promise to shape the healthcare landscape.
Understanding Medical Data Annotation
Medical data annotation is the process of labeling and categorizing various forms of medical data, including images, texts, and statistical records. By annotating this data, healthcare professionals can extract meaningful insights that can improve patient outcomes, streamline operations, and aide in research and development.
Types of Medical Data
The medical field comprises diverse types of data that require annotation:
- Images: Radiology images, MRIs, and CT scans are commonly annotated to identify diseases, monitor progression, or assess treatment effectiveness.
- Text: Clinical notes, patient records, and research papers are annotated to extract relevant information, enabling better decision-making.
- Statistical Data: Data derived from clinical trials and studies require annotation for analysis and interpretation.
The Importance of Medical Data Annotation
Accurate data annotation is vital in healthcare for several reasons:
Enhancing Machine Learning Models
With the rise of artificial intelligence in healthcare, annotated data is indispensable for training machine learning models. These models are utilized in:
- Diagnosis: AI systems trained on annotated data can assist radiologists in identifying tumors or abnormalities in medical imaging.
- Treatment Recommendations: Machine learning algorithms analyze vast datasets to recommend effective treatment plans tailored to individual patients.
- Predictive Analytics: Annotated data helps in forecasting disease outbreaks and assessing public health risks.
Promoting Research and Development
Enhanced research capabilities result from proper medical data annotation, facilitating:
- Collaborative Studies: Researchers can share annotated datasets to collaborate on studies that require extensive data.
- Clinical Trials: Annotated data is crucial in evaluating the efficacy and safety of new medical treatments.
- Medical Publications: Annotated text data ensures that research articles contain clear and concise findings accessible to the medical community.
Technologies Used in Medical Data Annotation
Several technologies and methodologies are employed in the medical data annotation process, including:
Automated Annotation Tools
With advancements in technology, automated tools can facilitate faster data annotation.
- Image Segmentation Tools: These tools help in accurately marking different regions in medical images, crucial for radiological evaluations.
- Natural Language Processing (NLP): NLP can automatically extract key information from clinical notes or research papers, making the annotation process more efficient.
- Annotation Platforms: Specialized software enables seamless collaboration among healthcare professionals and data annotators, ensuring consistency and accuracy.
Manual Annotation Techniques
While automation plays a significant role, human expertise is irreplaceable:
- Expert Review: Medical professionals review annotated data to ensure accuracy and relevance.
- Quality Control: Continuous monitoring and feedback loops ensure that the data annotation process meets high standards.
Challenges in Medical Data Annotation
Despite its significance, medical data annotation faces several challenges:
Data Privacy Concerns
With increasing digitization of medical records, data privacy has become a primary concern. Proper protocols must be in place to ensure that patient information is kept confidential during the annotation process.
Resource Intensive
Data annotation can be a time-consuming process requiring skilled professionals. Organizations must strike a balance between resource allocation and accuracy to maintain efficiency.
Standardization Issues
Inconsistent annotation formats can lead to data incompatibility. Establishing universal standards in medical data annotation is essential for improving interoperability across systems.
Future Trends in Medical Data Annotation
The field of medical data annotation is continuously evolving. Here are some trends to watch:
Integration of Advanced AI Techniques
Deep learning models will increasingly be applied to improve the accuracy of data annotation, enabling machines to learn from annotated data and improve over time.
Real-time Data Annotation
With the rise of telemedicine, real-time data annotation will become more important, facilitating immediate feedback for healthcare providers.
Collaborative Platforms
As healthcare professionals recognize the value of shared knowledge, collaborative platforms for data annotation will likely gain traction, enabling streamlined workflows and enhanced data access.
Conclusion: The Impact of Medical Data Annotation on Healthcare
In conclusion, medical data annotation is a fundamental component in advancing healthcare today. From enhancing machine learning models to facilitating impactful research, the implications of accurate and efficient data annotation are immense. As technology continues to develop, so will the potential for transforming the healthcare landscape, leading to improved patient outcomes and innovations in medical treatments. Embracing these changes will be vital for healthcare professionals and organizations aiming to stay at the forefront of the industry.
For more insights into healthcare innovations and advanced data solutions, visit keymakr.com.